Big data analytics has become increasingly important in recent years, and its role in entrepreneurial decision making cannot be underestimated. Big data refers to large volumes of unstructured and structured data that businesses collect from various sources, including social media, customer feedback, website traffic, and sales transactions. As companies are collecting more data than ever before, the importance of big data analytics is becoming more significant. The ability to analyze and interpret this vast amount of information has given entrepreneurs a competitive edge in their decision-making processes.
Entrepreneurial decision making involves identifying opportunities for growth and innovation while managing risk. It requires an understanding of the market trends, consumer behavior patterns, competition landscape, and other factors that impact business success. Big data analytics provides entrepreneurs with valuable insights into these areas by helping them identify patterns and trends that may not be visible through traditional methods. This article will explore the importance of big data analytics in entrepreneurship by examining how it helps companies understand consumer behavior, conduct market research and trend analysis, analyze competitors’ strategies, examine case studies from successful companies using big data analytics effectively, as well as discussing future benefits and limitations associated with this technology.
Definition of Big Data Analytics
The term ‘big data analytics’ refers to the process of analyzing large and complex data sets using advanced computational tools and techniques in order to extract meaningful insights. The importance of big data analytics in decision making cannot be overstated. In today’s world, businesses generate massive amounts of data from various sources such as social media, customer interactions, transactions, and digital footprints. Big data analytics enables organizations to turn this raw data into valuable insights that can inform crucial decisions.
Real-world applications of big data analytics are vast and varied. For instance, companies use it to optimize operations by identifying inefficiencies in their supply chain or production processes. They also use it for customer segmentation by analyzing behavioral patterns and demographics to target specific audiences with tailored marketing messages. Big data analytics is also used in fraud detection by identifying unusual transactions or activities that may indicate fraudulent behavior.
Big data analytics plays a critical role in decision-making across various industries. Its ability to analyze vast amounts of complex information is invaluable in providing businesses with an edge over their competition. With this understanding, we can now explore the importance of big data analytics in entrepreneurship – especially how it informs entrepreneurial decision-making processes through evidence-based insights rather than intuition alone.
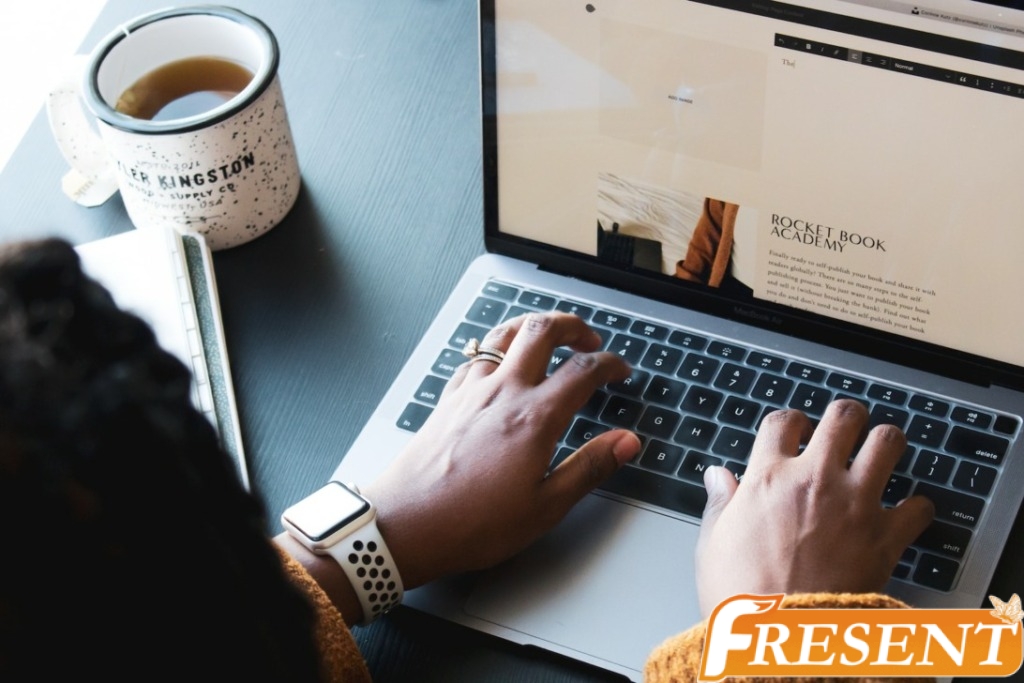
Importance of Big Data Analytics in Entrepreneurship
Significance of leveraging large datasets in the context of entrepreneurship cannot be overstated. Big data analytics has become a crucial tool for organizations to make informed decisions that impact their bottom line. Through advanced statistical techniques, businesses can identify patterns and trends that would have otherwise remained hidden. This information can then be used to optimize processes, reduce costs, improve customer experience, and create new products/services.
One of the potential applications of big data analytics in entrepreneurship is its ability to provide insights into consumer behavior. By collecting and analyzing vast amounts of data on consumer preferences, purchasing habits, and social media activity, businesses can gain a better understanding of what drives customer decision-making. This information can inform marketing strategies, product development efforts, and pricing decisions. In this way, big data analytics has a significant impact on decision making by enabling entrepreneurs to make more informed choices that are grounded in empirical evidence rather than intuition.
Big data analytics has revolutionized the way entrepreneurs approach decision making. Its ability to process large amounts of complex data quickly and accurately provides valuable insights into business operations that were previously unattainable. By leveraging these insights effectively, entrepreneurs can gain a competitive edge in their industry while providing customers with products or services that meet their needs. Understanding consumer behavior is just one step towards achieving success through big data analysis; the next section will explore this topic further by examining how businesses use this information to develop targeted marketing campaigns and tailored product offerings.
Understanding Consumer Behavior
Understanding consumer behavior is a crucial aspect of entrepreneurship, as it enables businesses to tailor their products or services to meet the needs and preferences of their customers. To achieve this, data collection and analysis play a significant role in identifying customer preferences and trends. By utilizing big data analytics tools, entrepreneurs can gain insights into consumer behavior patterns that are essential for customizing products or services that align with the needs of their target audience.
Data Collection and Analysis
Applying a magnifying glass to the data collection and analysis process can yield valuable insights for entrepreneurs, helping them make informed decisions. To achieve accurate data collection, it is crucial to ensure that the data collected is reliable, valid, and relevant to the research objectives. Data accuracy can be achieved by using appropriate sampling methods, ensuring that the data collected is free from errors or biases, and ensuring that data sources are credible. It is also important to interpret the collected data correctly to obtain meaningful insights into consumer behavior patterns. By analyzing customer preferences, behaviors, and demographics entrepreneurs can better understand their market segment and tailor their product or service offerings accordingly.
Furthermore, big data analytics tools help in identifying trends and patterns in large datasets that would otherwise go unnoticed using traditional statistical techniques. By leveraging these tools’ capabilities such as machine learning algorithms and natural language processing techniques, businesses can extract useful information from unstructured datasets like social media interactions or customer feedback comments. The insights gained through these sophisticated analytical techniques provide entrepreneurs with valuable information about consumer sentiments towards their products/services leading to better decision making. Understanding how customers interact with products/services provides an opportunity for businesses to identify areas for improvement or potential new revenue streams based on what drives customer satisfaction levels. In summary, accurate data collection coupled with proper interpretation enables businesses of all sizes to gain actionable insights into their target markets leading to more informed entrepreneurial decision-making processes where customer needs are prioritized over anything else.
Identifying Customer Preferences
Identifying customer preferences is a critical step for businesses seeking to tailor their products or services to meet the needs of their target market. One of the ways that big data analytics can assist with this process is through customer segmentation. By analyzing large amounts of data, businesses can group customers into distinct categories based on demographics, purchasing behaviors, and other relevant factors. This allows them to create targeted marketing campaigns and offer personalized products or services that are more likely to resonate with each segment.
In addition to segmentation, big data analytics also enables product personalization. By tracking customer interactions with a business’s website, social media accounts, and other channels, companies can gain insights into individual preferences and interests. They can then use this information to customize offerings for each customer, providing a more unique experience that increases loyalty and brand advocacy. With these tools at their disposal, entrepreneurs can make more informed decisions about how best to serve their target market in an increasingly competitive landscape. The next section will explore the ways in which businesses can implement these strategies for customizing products or services based on big data analysis results.
Customizing Products or Services
After identifying customer preferences, the next step for entrepreneurs is to customize their products or services. Customization benefits businesses by increasing customer satisfaction and loyalty, as it allows them to tailor their offerings to individual needs and interests. With the help of big data analytics, businesses can use personalized marketing strategies that target specific customers with relevant messaging and promotions.
Personalized marketing strategies are effective because they allow businesses to create a more meaningful connection with their customers. By analyzing customer data, businesses can understand their behaviors and preferences and deliver targeted messages that resonate with them on a personal level. This approach not only increases sales but also helps build long-term relationships with customers who feel understood and valued by the business.
Moving forward into market research and trend analysis, entrepreneurs must continue to leverage big data analytics to identify emerging trends in consumer behavior.
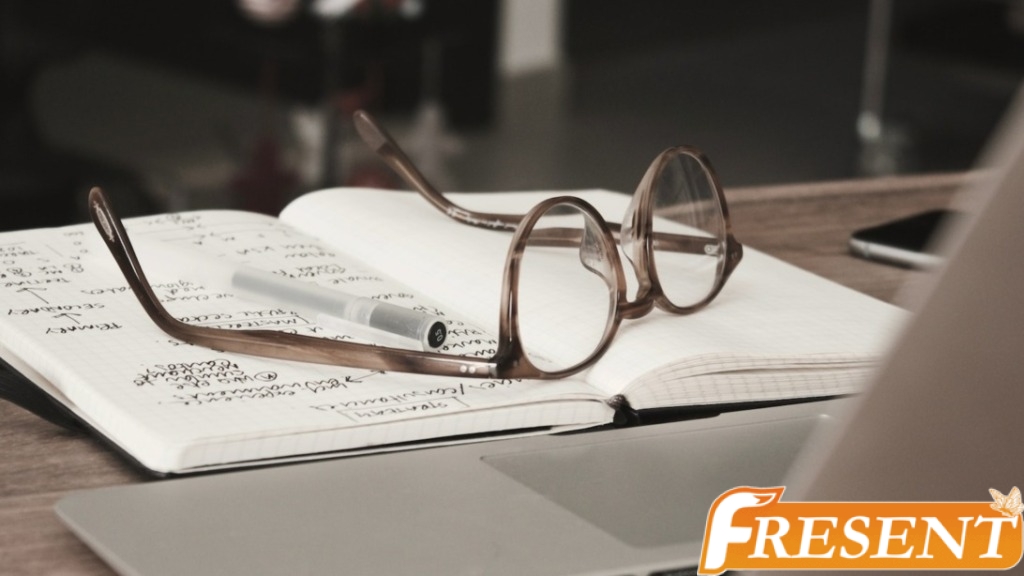
Market Research and Trend Analysis
Market research and trend analysis play a crucial role in providing entrepreneurs with an accurate understanding of the current market landscape and consumer behavior. Social media has become one of the most effective tools for conducting market research. Entrepreneurs can use social media platforms to gather data on consumer preferences, opinions, and behaviors. Consumer surveys are another popular method used to collect market data. By analyzing survey responses, entrepreneurs can gain insights into customer needs and tailor their products or services accordingly.
Industry reports also provide a wealth of information that entrepreneurs can use to inform their decision-making. These reports offer detailed analyses of industry trends, competitive landscapes, and consumer behavior patterns. Data visualization tools are often used to present this information in a visually appealing way that is easy to comprehend. Entrepreneurs can use this information to identify gaps in the market or areas where they can differentiate themselves from competitors.
Conducting thorough market research and trend analysis is essential for entrepreneurial success. By leveraging social media platforms, conducting consumer surveys, reviewing industry reports, and using data visualization tools, entrepreneurs can gain valuable insights into the current marketplace. This knowledge allows them to make informed decisions about product development, marketing strategies, pricing models, and more. In the next section on competitor analysis…
Competitor Analysis
Examining the strategies and tactics of competitors can provide entrepreneurs with valuable insights into their own industry and potential areas for improvement. By conducting a competitor analysis, business owners can identify key players in their field, determine their strengths and weaknesses, and develop strategies to differentiate themselves from the competition. Competitor analysis tools such as SWOT analysis techniques allow entrepreneurs to evaluate the internal strengths and weaknesses of their own company in comparison to those of their competitors.
SWOT (Strengths, Weaknesses, Opportunities, Threats) analysis is an effective tool used in competitor analysis. This technique helps businesses identify the strengths and weaknesses of their own company as well as those of their competitors. The strengths and weaknesses are assessed internally while opportunities and threats are evaluated externally. Through this process, businesses can gain insight into how they can improve their operations or capitalize on market trends that other companies have failed to address.
In addition to SWOT analysis techniques, there are other competitor analysis tools that entrepreneurs can utilize to stay ahead of the game. These include benchmarking against industry leaders, monitoring customer reviews for both your company’s products/services as well as those offered by competitors’, analyzing pricing strategies across various brands/products within your niche etc. By understanding what sets them apart from others in the market through these analytical tools , entrepreneurs can make informed decisions about future product development or marketing campaigns without relying solely upon intuition or guesswork.
As we move onto data collection and management section it should be noted that utilizing competitor analysis tools is essential but it’s equally important to gather accurate data before drawing any conclusions based on said analytics . Therefore proper collection , organization ,and interpretation of data plays a critical role in making entrepreneurial decisions backed by big data analytics .
Data Collection and Management
The collection and management of big data is essential for entrepreneurial decision-making. Effective data collection methods and techniques must be employed to ensure that relevant information is obtained from various sources such as social media, online transactions, and customer feedback. Additionally, proper data management strategies should be in place to organize, store, analyze, and interpret the collected data accurately. Lastly, ensuring the security of the collected data is crucial to protect sensitive information from unauthorized access or cyber-attacks.
Sources of Big Data
Various reservoirs of information can be explored to gain insights into big data, and entrepreneurs have a wealth of options at their disposal. These sources include customer interactions, social media activities, IoT sensors, and machine-generated data. Customer interactions with businesses such as feedback on products or services can provide valuable insights that help entrepreneurs tailor their offerings for better results. Social media is another critical source of big data because conversations on various platforms often reflect people’s thoughts about different aspects of life.
A challenge faced when sourcing big data is the sheer volume of information available across these sources. It can be overwhelming for entrepreneurs to determine which datasets are most relevant to their business objectives and how best to extract value from them. However, with appropriate analysis techniques in place, companies can use this vast quantity of data to make informed decisions about everything from product development and marketing strategies to supply chain optimization and resource allocation. The next section will discuss some important data management techniques that enable organizations to harness the power of big data effectively.
Data Management Techniques
Effective management of data is crucial for organizations to derive valuable insights and make informed decisions based on the vast amount of information available. Data quality plays a significant role in data management techniques as the accuracy and consistency of data are essential for decision-making processes. Inaccurate or inconsistent data can lead to incorrect conclusions, which can result in poor business decisions. Therefore, organizations must have effective methods for ensuring that their data is accurate and consistent.
Data governance is another critical aspect of managing big data effectively. It involves establishing policies, procedures, roles, responsibilities, and standards for managing an organization’s information assets. By implementing robust data governance practices, organizations can ensure that their big data initiatives align with business objectives while also maintaining compliance with regulatory requirements. Effective data governance helps organizations achieve better decision-making capabilities by providing transparency into how the organization manages its information assets. This results in more reliable and trustworthy insights from big data analytics that ultimately support better decision-making processes. As such, it is crucial to ensure proper handling of sensitive information by discussing the importance of secure storage facilities in the next section about ‘importance of data security.’
Importance of Data Security
Data security is a critical component in safeguarding an organization’s information assets and preventing unauthorized access to sensitive data. As the saying goes, ‘prevention is better than cure.’ Organizations need to ensure that they have implemented robust cybersecurity measures to protect their data from external cyber threats. One effective way of safeguarding data is through data encryption, which involves converting plain text into ciphertext using complex algorithms. This process ensures that only authorized personnel with the decryption key can access sensitive information.
To further enhance data security, organizations should also implement additional cybersecurity measures such as firewalls, intrusion detection systems, and anti-malware software. These measures help prevent cyberattacks by detecting and blocking malicious activities before they cause harm. Additionally, organizations must provide regular training to staff on best practices for information security to minimize potential human errors that could compromise the integrity of their data.
Ensuring proper data security is crucial for any organization that wants to leverage big data analytics in decision making successfully. By implementing robust cybersecurity measures such as encryption and other protective technologies alongside providing proper employee training on good practices for information security, companies can mitigate risks associated with unauthorized access or loss of their valuable assets while enjoying benefits of big-data analysis without fear or hesitation. The next section will discuss how entrepreneurial decision-making processes benefit from adequate processing and analysis techniques that take full advantage of available data sources while minimizing risks associated with poor management habits like inadequate storage infrastructure or unsecured networks vulnerable attackers who seek personal gain at others’ expense.
Data Processing and Analysis
The Subtopic of Data Processing and Analysis is concerned with the techniques used to prepare and analyze data in order to obtain meaningful insights. Data pre-processing involves cleaning, transforming, and integrating data from various sources to ensure accuracy and consistency. Once the data is prepared, a range of analysis techniques can be employed such as regression analysis, clustering, or machine learning algorithms. The results of these analyses are often visualized through charts, graphs or other graphical representations that make it easier for decision-makers to understand patterns and trends in the data.
Data Pre-processing
Pre-processing of data is a critical stage in the big data analytics process that lays the foundation for accurate and reliable insights. Data cleaning is necessary to remove inconsistencies, errors, and irrelevant information that can impact the integrity of analysis results. It involves identifying missing values, duplicates, and formatting issues to ensure consistency across all data elements. Outlier detection is another important aspect of pre-processing as it enables identification and removal of extreme values that do not conform to typical patterns.
To engage the audience, here are some key considerations during data pre-processing:
- Ensure adequate documentation of all changes made during pre-processing
- Use statistical methods to identify outliers and missing values
- Standardize variables to enable meaningful comparisons between different datasets
- Apply appropriate scaling techniques to normalize variable ranges
- Consider using automated tools for more efficient and accurate pre-processing.
Moving on to data analysis techniques, these rely heavily on the quality and reliability of preprocessed data. Therefore, it is essential to invest sufficient time into this crucial stage before proceeding further with analytical tasks.
Data Analysis Techniques
One of the fundamental techniques used in analyzing large datasets is exploratory data analysis (EDA), which involves visualizing and summarizing data to gain insights into underlying patterns and relationships. EDA can reveal trends, anomalies, correlations, and outliers that may be hidden in raw data. Regression analysis is one of the most common methods used for predicting a target variable based on one or more predictor variables. It helps entrepreneurs understand the relationship between different variables and how they affect each other. Predictive modeling is another technique that uses statistical algorithms to identify patterns in historical data and make predictions about future outcomes.
Cluster analysis and data mining are also essential techniques for identifying groups or clusters within a dataset that share similar characteristics. These methods help entrepreneurs segment their customer base or market into distinct groups with different needs, preferences, behaviors or purchasing patterns. This information can then be used to tailor marketing strategies accordingly. Visualizing data using charts, graphs, histograms, heat maps or other graphical representations is an important step in analyzing big data because it allows entrepreneurs to communicate complex ideas more clearly and effectively than just presenting raw numbers alone. By examining various visualization techniques alongside statistical models such as regression analysis and predictive modeling as well as clustering methods like cluster analysis and data mining – entrepreneurs can make informed decisions based on empirical evidence that supports their business goals while reducing risk associated with uncertainty regarding potential outcomes of questionable business decisions due to lack of accurate information about their customers’ behavior patterns over time.
Visualization of Data
Visualizing data through various graphical representations and statistical models is a critical aspect of analyzing large datasets for gaining insights into underlying patterns and relationships, which can inform strategic decision-making for businesses. Data visualization techniques help to transform raw data into meaningful and useful information that can be easily interpreted by decision-makers. Infographic design principles have become increasingly important in recent years because they allow complex data to be presented in a visually appealing manner, making it easier for users to understand patterns and trends.
Effective data visualization requires careful selection of the appropriate type of graph or chart based on the dataset’s characteristics. Pie charts, bar graphs, line graphs, scatter plots, heat maps, treemaps are some common types of visualizations used in business analysis. The main goal is to present the data accurately while highlighting essential features such as outliers or trends that can lead to actionable insights. By incorporating effective infographic design principles such as using color schemes or selecting appropriate fonts and layout designs, businesses can create visually appealing visualizations that enhance communication with stakeholders.
The field of big data analytics continues to evolve rapidly with technological advancements such as machine learning and artificial intelligence playing an increasingly significant role in processing vast amounts of structured and unstructured data sets.
Machine Learning and Artificial Intelligence
The integration of machine learning and artificial intelligence technologies in big data analytics has significantly enhanced the accuracy and efficiency of entrepreneurial decision making. These advanced technologies allow entrepreneurs to analyze vast amounts of data quickly and accurately, providing insights that were previously impossible to obtain. By leveraging machine learning algorithms, businesses can identify patterns and trends in their data, which can help them make informed decisions about product development, marketing strategies, and customer engagement.
However, the use of machine learning and AI in big data analytics also raises important ethical questions. For example, some applications may violate privacy laws or compromise sensitive information. It is essential for entrepreneurs to be mindful of these concerns when implementing new technologies into their business processes. Real-world examples of ethical violations include discriminatory practices by hiring algorithms or bias in loan approval systems.
Despite these challenges, the benefits of integrating machine learning and AI into big data analytics outweigh the risks if done correctly. Predictive analytics is one area where these technologies are particularly useful. By analyzing past consumer behavior patterns using predictive models, businesses can forecast future market trends with greater accuracy than ever before. This enables entrepreneurs to make better-informed decisions about everything from inventory management to pricing strategies – ultimately leading to increased profitability and growth opportunities for their businesses.
Predictive Analytics
Predictive analytics revolutionizes the way businesses forecast market trends, providing entrepreneurs with valuable insights to make informed decisions and drive growth. With big data at their disposal, predictive analytics can identify patterns in customer behavior, sales data and social media activity to predict future outcomes. Here are three ways predictive analytics is being applied:
- Customer Relationship Management: Predictive analytics can help entrepreneurs develop a better understanding of their customers by analyzing past purchase history and preferences. This information can be used to improve customer service, target marketing campaigns and ultimately increase sales.
- Supply Chain Optimization: Predictive analytics can also be used to optimize supply chain management by predicting demand for specific products or components, identifying potential bottlenecks in the production process and improving inventory management.
- Fraud Detection: Predictive analytics has become an essential tool for identifying fraudulent activities in financial institutions such as banks or insurance companies. By analyzing transactional data and detecting unusual patterns of behavior, predictive models can help prevent fraud before it occurs.
Despite its many benefits, there are limitations and challenges associated with using predictive analytics in entrepreneurial decision making. One major challenge is ensuring the accuracy of the models used for prediction as they heavily rely on historical data that may not always reflect current market conditions accurately. Additionally, privacy concerns arise when dealing with sensitive personal information collected from customers during analysis.
Despite its limitations and challenges, predictive analytics remains an essential tool for entrepreneurs seeking to gain a competitive edge in today’s fast-paced business environment. The implementation of big data analytics requires careful consideration of various factors such as technology infrastructure, talent acquisition and regulatory compliance to ensure successful adoption into daily operations.
Implementation of Big Data Analytics
The implementation of Big Data Analytics involves setting up a comprehensive system that can handle large amounts of data, hiring a knowledgeable data analyst to manage the system and perform analyses, and addressing challenges that may arise during the implementation process. Setting up an effective Big Data Analytics system requires identifying the right hardware and software tools needed for data storage, processing, and analysis. Hiring a qualified data analyst with expertise in statistical analysis, programming languages such as Python or R, and experience working with databases is essential for ensuring accurate interpretation of results and successful implementation of Big Data Analytics.
Setting Up a Big Data Analytics System
Implementing a comprehensive big data analytics system requires strategic planning, efficient data collection methods, advanced analytical tools, and a skilled workforce. The first step in setting up such a system is to assess the cost implications and technical requirements. This involves identifying the necessary hardware and software components, including servers, storage devices, and analytical tools. Additionally, it is important to consider the costs associated with hiring personnel who are qualified to manage and analyze large volumes of data.
Once the technical specifications have been determined, the next step is to ensure that efficient data collection methods are in place. This involves selecting appropriate data sources and designing effective data collection processes that will yield high-quality information for analysis. In addition to technical considerations, it is important to invest in training programs that will equip employees with the necessary skills to work effectively within this new system. Having established an effective big data analytics system, companies can then focus on hiring a skilled data analyst who can help them make informed decisions based on the insights generated by their new technology infrastructure.
Hiring a Data Analyst
Hiring a skilled data analyst is crucial for companies seeking to derive meaningful insights from their vast data resources. Finding candidates with the right qualifications and experience can be challenging, but there are several strategies that can help. One approach is to look for individuals who have completed relevant education or training programs in data analytics, statistics, or computer science. Additionally, companies may search for candidates who have worked in related industries or functions and have experience working with similar datasets.
The interview process is an important step in hiring a data analyst. Companies should prepare specific questions that test candidates’ technical skills as well as their ability to communicate complex findings to non-technical stakeholders. It is also valuable to ask about previous projects and work experiences to gain insight into how the candidate thinks about problems and approaches solutions. Ultimately, hiring the right data analyst can make a significant impact on a company’s ability to leverage big data analytics for decision making.
Transition: While finding and hiring the right talent is essential for implementing big data analytics successfully, it is not without its challenges.
Challenges in Implementing Big Data Analytics
Organizational readiness and cultural alignment are significant hurdles that companies need to overcome when adopting big data analytics. One challenge is ensuring data integrity throughout the entire data lifecycle, from collection to analysis. Organizations must establish strict protocols for data ingestion and processing, as well as implement quality control measures to ensure accuracy and completeness of the data. This requires a shift in mindset from traditional decision-making processes based on intuition or historical trends towards evidence-based decision-making fueled by reliable datasets.
Another challenge is scalability, which refers to the ability of an organization’s infrastructure, resources, and people to handle increasingly large amounts of data. Scaling up can be expensive and time-consuming, requiring upgrades in hardware and software capabilities as well as retraining personnel on new tools and techniques. Additionally, organizations must consider privacy laws and regulations when implementing big data analytics initiatives since sensitive information may be exposed during the process.
Despite these challenges, big data analytics provides organizations with valuable insights for making informed business decisions. Case studies offer examples of successful implementation strategies that have resulted in increased productivity, profitability, and customer satisfaction.
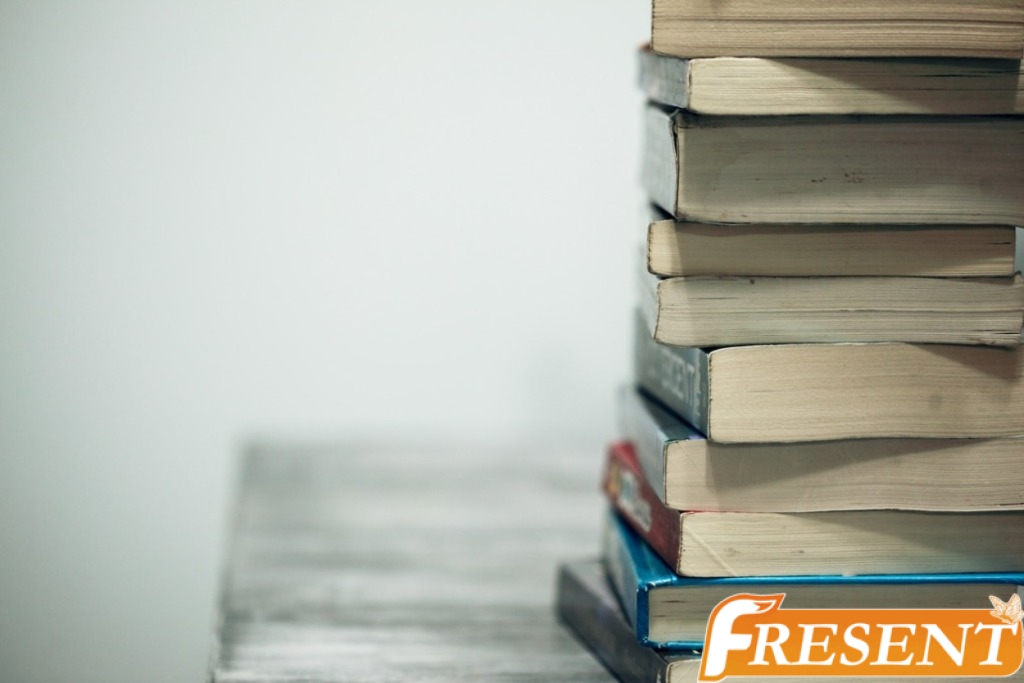
Case Studies
Exemplifying the practicality of big data analytics in entrepreneurial decision making, case studies offer valuable insights into how businesses can optimize their operations and gain a competitive edge. Real life examples demonstrate the impact of data-driven decision making on various industries, including healthcare, retail, finance and manufacturing. For instance, Amazon’s recommendation engine uses customers’ purchase history to provide personalized product suggestions. This has not only increased customer loyalty but also boosted sales by up to 35%.
Similarly, GE Healthcare has been using machine learning algorithms to analyze vast amounts of medical data and improve patient outcomes. By identifying patterns and predicting potential health risks early on, doctors can take proactive measures that prevent complications and save lives. Another example is Walmart’s use of big data analytics to optimize their supply chain management system. The company’s predictive analytics technology helps them anticipate demand for products and ensure timely delivery.
These case studies highlight the potential for big data analytics to revolutionize business operations across different sectors. As more companies adopt these technologies, we are likely to see even more significant changes in the way entrepreneurs make decisions. In the next section about ‘future of big data analytics,’ we will explore emerging trends in this field and how they can influence entrepreneurial strategy going forward.
Future of Big Data Analytics
The ever-evolving landscape of technology and the exponential growth of data sources have transformed the way businesses operate. Big data analytics has emerged as an essential tool in understanding consumer behavior, identifying market trends, and improving operational efficiencies. The future of big data analytics presents a range of opportunities for organizations to gain valuable insights into their operations, customers, and competition.
Innovations in big data analytics have made it easier to analyze large datasets quickly. With advances in machine learning and artificial intelligence, businesses can now extract valuable insights from vast amounts of structured and unstructured data. Predictive analytics models can help companies forecast trends and identify new business opportunities that would otherwise go unnoticed.
However, the future of big data analytics is not without its challenges. As businesses collect more information about their customers, they must also ensure that they comply with privacy regulations such as GDPR or CCPA. Additionally, there is a shortage of skilled professionals who are capable of working with advanced analytical tools effectively. Despite these challenges, the benefits of big data analytics outweigh any potential drawbacks.
As organizations continue to invest in big data analytics technology and workflows, they are well-positioned to leverage their insights to drive better decision-making processes across various departments ranging from marketing through supply chain management. By using predictive modeling techniques to anticipate customer needs or optimizing production schedules based on real-time demand signals – companies will be able to achieve enhanced outcomes at lower costs than previously possible while simultaneously enabling better decision-making within all aspects throughout their organization without relying solely on intuition or gut feelings alone- ultimately driving greater competitive advantage over time!
Benefits of Big Data Analytics
One significant advantage of utilizing data analytics tools is the ability to gain valuable insights into consumer behavior and market trends. With access to vast amounts of data, entrepreneurs can make informed decisions about product development, marketing strategies, and customer targeting. By analyzing patterns in consumer behavior and identifying emerging trends, businesses can stay ahead of the competition and remain relevant in an ever-changing market.
Data-driven decision making also allows businesses to optimize their operations and increase efficiency. By tracking key performance indicators (KPIs) such as sales figures, inventory levels, and website traffic, entrepreneurs can identify areas for improvement and implement targeted solutions. This not only saves time and resources but also helps businesses achieve a competitive advantage by operating more efficiently than their rivals.
In addition to improving operational efficiency, big data analytics also enables entrepreneurs to personalize their offerings and provide better customer experiences. By collecting data on individual customers’ preferences, purchasing habits, and feedback, businesses can tailor their products or services accordingly. This enhances customer satisfaction while increasing the likelihood of repeat business. However, despite these benefits, there are limitations to big data analytics that must be considered when utilizing this approach for decision making purposes.
As we’ll explore in the subsequent section about limitations of big data analytics , it’s important for entrepreneurs to recognize that while big data provides valuable insights into consumer behavior and market trends; it isn’t a panacea for all business challenges. Ultimately successful utilization requires careful consideration of its potential impact on organizational processes as well as recognition of its limitations in terms of accuracy shortcomings due to incomplete datasets or other factors such as biases or contextual considerations within different markets or industries where certain approaches may not apply equally well across all domains due either technical barriers present at the current time or human factors influencing how people interact with technology-based solutions over long periods over time leading them being less effective than hoped initially envisioned upon first implementation efforts being undertaken originally planned out initially before implementing changes based solely on raw numerical metrics without taking into account other important factors that influence business outcomes.
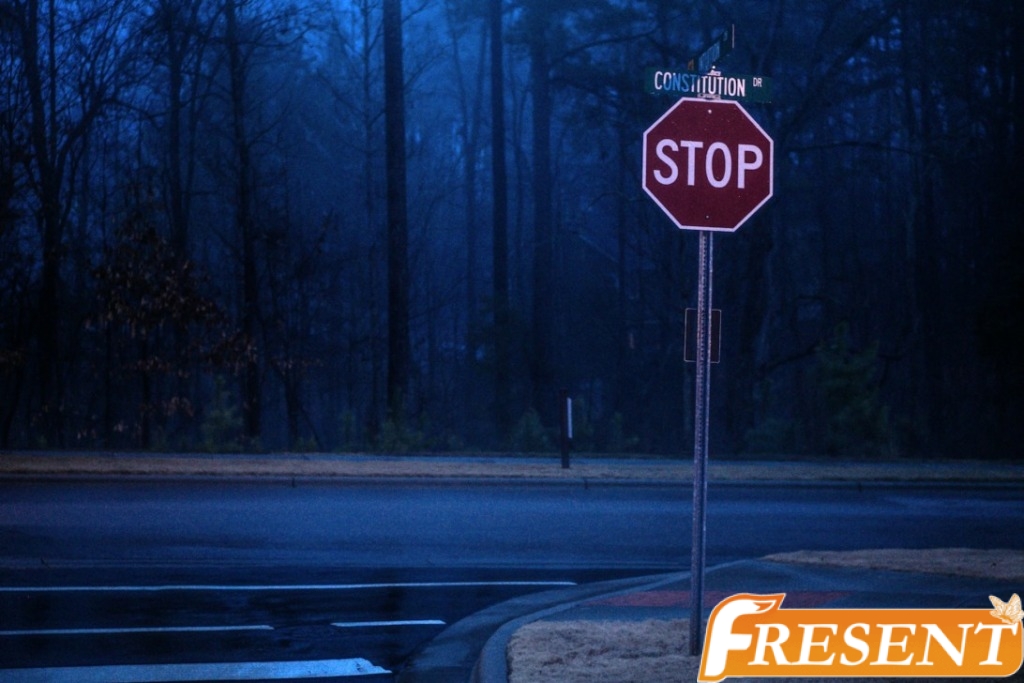
Limitations of Big Data Analytics
The use of big data analytics in decision making has revolutionized the way businesses operate. However, there are concerns about data privacy and security that have become a growing concern for individuals and organizations alike. Moreover, there is also a potential for bias and misinterpretation of results due to limitations in data collection methods. Thus, it is important to consider these limitations when using big data analytics to ensure that the insights gained from such analysis are accurate and reliable.
Data Privacy Concerns
Data privacy concerns pose a significant challenge for entrepreneurs utilizing big data analytics in their decision-making processes. Data privacy regulations and ethical considerations are among the primary issues that entrepreneurs need to address when leveraging big data analysis. As more personal information is collected, stored, and analyzed, there is an increasing risk of data breaches, identity theft, and surveillance. These risks can undermine consumer trust and jeopardize business reputation.
Entrepreneurs must also be mindful of the ethical implications of big data analytics. They should ensure that they are transparent about their methods of collecting and analyzing data to avoid any potential harm or exploitation to individuals or groups involved in their studies. Additionally, entrepreneurs must be aware of any legal obligations associated with the collection and use of personal information. In sum, while big data analytics offers tremendous opportunities for entrepreneurial growth and development, it is important that these opportunities are leveraged responsibly with due consideration given to data privacy regulations and ethical considerations. This will help mitigate risks associated with bias and misinterpretation which we will discuss in the subsequent section.
Bias and Misinterpretation
Bias and misinterpretation are significant challenges that must be addressed in utilizing the insights gained from big data analysis. One of the main sources of bias is the uneven representation of certain groups within the data. This can lead to overgeneralization or underestimation of particular trends, which may result in inaccurate predictions or conclusions. Another important factor contributing to bias is the presence of implicit assumptions or preconceptions that influence how data is collected and analyzed. For example, if a researcher assumes that a particular demographic group behaves in a certain way, this assumption may unconsciously shape their interpretation of the data.
To overcome biases and ensure accurate interpretation of big data analytics, there are several techniques that can be employed:
- Employing diverse teams with different perspectives
- Using random sampling to ensure representative samples
- Questioning assumptions and being aware of unconscious biases
- Conducting sensitivity analyses to explore alternative interpretations
- Utilizing transparent and reproducible methods for analyzing data
While these techniques cannot eliminate all potential sources of bias, they can help minimize their impact on decision-making processes based on big data analytics.
Moving forward, it’s important to recognize both the potential benefits and limitations of using big data analytics in entrepreneurial decision making.
Limitations of Data Collection
One important consideration when utilizing big data analytics is the challenge of collecting comprehensive and accurate data. While there are numerous sources of information available, not all might be relevant or reliable for a particular analysis. Incomplete or inaccurate data can lead to flawed conclusions and misguided decisions that could have serious implications for entrepreneurial ventures.
Implications of incomplete data can range from missing key variables to biased samples, both of which can distort the accuracy of big data analytics. Additionally, challenges in data accuracy can arise due to measurement error, inconsistencies in coding and labeling, and differences in interpretation across observers. To address these limitations, it is crucial for entrepreneurs to carefully evaluate their sources of information, validate their datasets through cross-checking with other sources or methods, and acknowledge any potential limitations or biases in their analyses. By doing so, they can ensure that their decision-making process is based on sound evidence rather than incomplete or inaccurate assumptions about the market or customer behavior.
Conclusion
Big data analytics has become an essential tool for modern entrepreneurs in making informed business decisions. By leveraging the vast amounts of data generated by consumers, businesses can gain valuable insights into their behavior and preferences. This information is critical in understanding market trends, identifying competitors’ strengths and weaknesses, and developing innovative strategies to stay ahead of the competition.
However, while big data analytics offers tremendous benefits to entrepreneurs, it’s crucial to acknowledge its limitations. The accuracy of the data collected is dependent on a range of factors such as sample size, collection methods, and quality control measures. Additionally, interpreting this data requires specialized skills that not all entrepreneurs possess.
In conclusion, while big data analytics has revolutionized entrepreneurial decision-making by providing unprecedented access to consumer insights and trend analysis, it’s not a panacea for all business problems. Entrepreneurs must be mindful of its limitations while maximizing its potential benefits through careful analysis and interpretation of the available data. Ultimately, success lies in striking a balance between intuition-based decision-making and evidence-based approaches that leverage big data analytics.